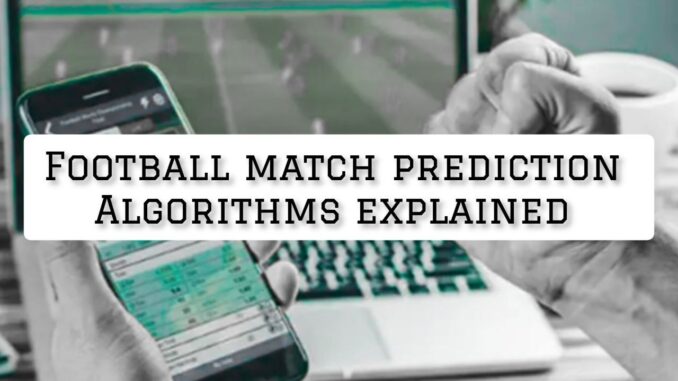
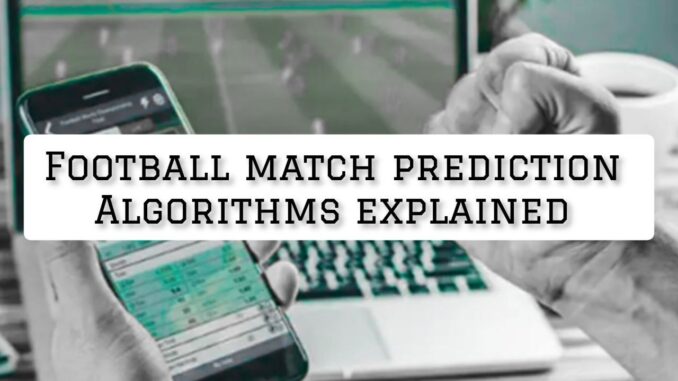
When it comes to that Predict football matcheswhat if I told you a challenging one algorithm maybe have better chances than an experienced fan? While experience and gut feeling play a role, today’s football prediction algorithms utilize massive amounts of data and go deeper and more precise than anything humanly possible. But what are these algorithms really doing behind the scenes to crack the code of game results?
This is where things get interesting. At its core, a football match prediction algorithm is about recognizing patterns. Think about how a detective analyzes clues at a crime scene. To an algorithm, these “hints” are statistics.
A predictive model sifts through player stats, team records, historical matchups, even weather conditions and injury updates. These algorithms are so detail-oriented that they take into account the team’s previous performance in similar circumstances, such as away games or dealing with player absences.
It’s not just about wins and losses; It’s about the context of every game, every move, every goal. You are reading a novel in numbers.
Imagine two soccer teams competing against each other, and the algorithm breaks down each team’s probability of winning based on countless inputs or “features.” This includes the number of goals scored, the strength of the defense, the current form and the direct record of the individual teams.
Algorithms first analyze past games to create a baseline and identify the attributes that lead to victory for each team. For example, some teams have made a strong start in the first half but are struggling to maintain the pace.
Others may be able to make better use of their scoring opportunities when playing at home. This level of statistical precision would put even the most die-hard fan to shame.
But algorithms don’t just play a single game with the data – they evolve. This is where machine learning comes into play, allowing these algorithms to continually learn from new data. Each match provides the algorithm with new information so it can refine its predictions for next time.
For example, let’s say a team just signed a star forward who has a high goal average. The algorithm adjusts and weights its calculations to take into account the potential impact of this new player. If a star player is sidelined due to injury, the model’s output changes accordingly and its prediction is recalibrated based on that absence. This self-learning ability is like a constantly updated encyclopedia of the football universe.
And it doesn’t stop there. Some of the most advanced prediction algorithms are based on machine learning models like neural networks. Neural networks mimic the neural connections of the human brain and can thus recognize complex, non-linear relationships in data.
That’s why they don’t forecast based solely on “if this, then that” logic. They look for levels of data interactions that we would never discover. For example, if they find a connection between rainy weather and a lower score for a particular team, that will be taken into account.
These networks can even pick up on subtleties such as the influence of crowd noise at away games or the motivation boost that winning streaks provide. When an algorithm has this level of sophistication, you know it goes beyond anything a spreadsheet could capture.
However, prediction algorithms don’t always get everything right. Football is notoriously unpredictable. Sudden red cards, accidental goals, weather changes, unexpected player injuries – none of this can be perfectly quantified. An algorithm could predict that a particular team will dominate possession and create more chances to score, but it cannot predict that a defender will slip in the mud or a referee will make a questionable decision.
What it can do, however, is understand probabilities in a way that takes these uncertainties into account. Most algorithms output probabilities, not certainties. They don’t say “this team.” will win”; They say that based on the data at hand, there is a 70% chance of Team A winning.
Some may say this approach takes the magic away from the game, but for many it adds an extra layer of thrill. Imagine watching a game and knowing that an algorithm predicted a narrow 2-1 win. Suddenly every movement feels more intense, every missed opportunity more meaningful. It’s as if the algorithm adds an extra layer of drama, revealing hidden dynamics that most fans wouldn’t otherwise appreciate.
And while algorithms have had an impact, they have not yet replaced human intuition. There is something unique about the “hunch” of a lifelong fan that no machine has ever been able to replicate.
Some of the most remarkable upsets in football history – think underdog victories in tournaments or unexpected comebacks – are moments that defy data. For fans, these are the most exciting moments that make football more than just a game.
As football prediction algorithms continue to evolve, they are getting better at “understanding” the game. It is now common for large clubs to employ data analysts whose sole job is to support the team’s playing model. They use similar algorithms for lineup optimization, game tactics and even recruiting.
Football has entered an age where science and sport are intertwined, a fusion of data, technology and athleticism that is revolutionizing the way teams prepare and play. Whether you believe in algorithmic predictions or a traditionalist who prefers intuition, one thing is certain: the algorithm is here to stay.