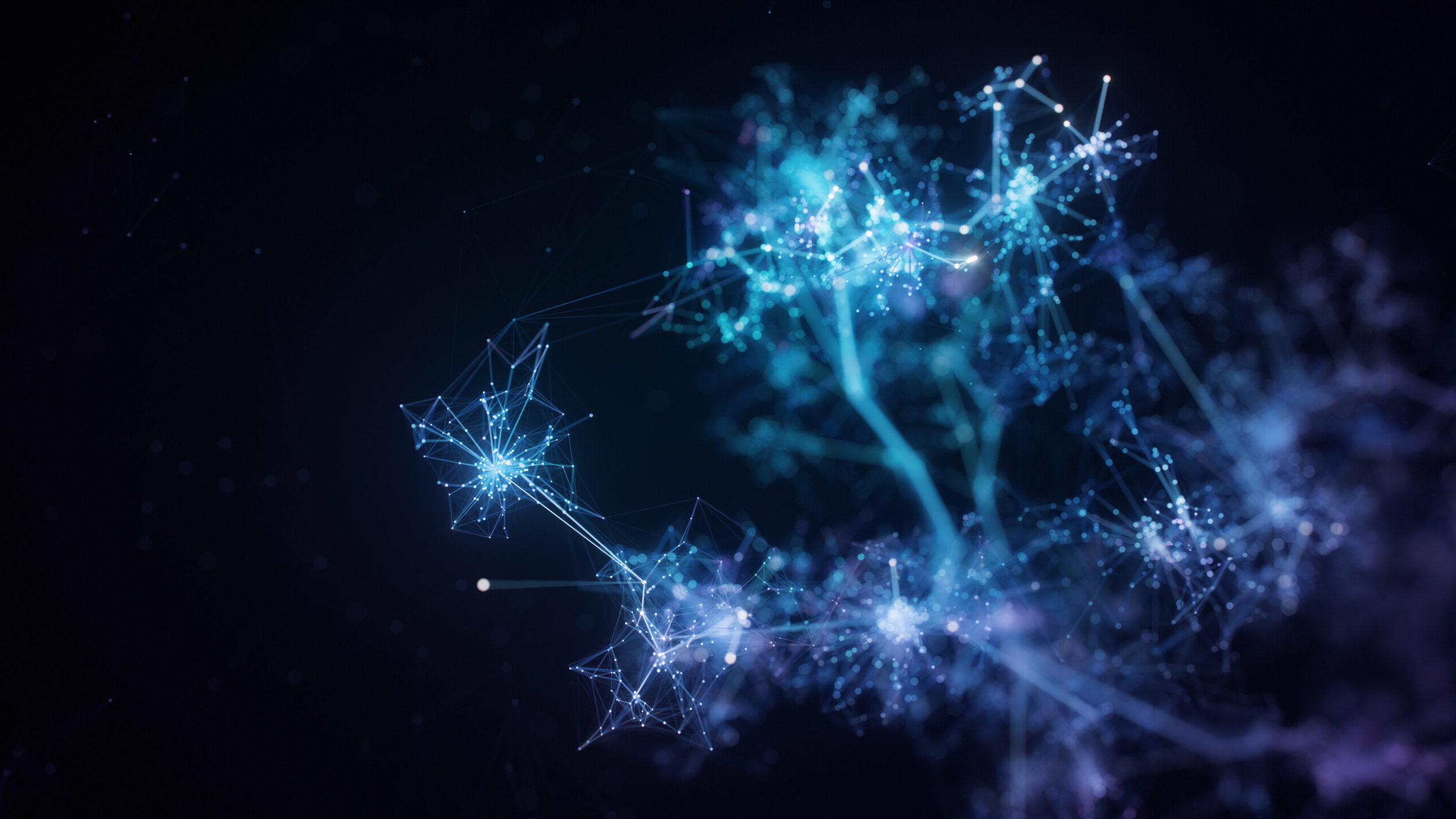
The rise of enterprise AI
AI adoption in banks has been supercharged by generative AI. But is this just another short-lived and overhyped technology? Or is it truly the start of a new revolution in financial services?
The financial services industry has had several similar moments in the past decade, yet not all of them have resulted in true transformation. For example, blockchain in 2017 led many to believe it would completely revolutionize banking, but nearly a decade later, it remains a theoretical interest.
A more recent example is the metaverse, which also generated significant excitement but has yet to see substantial adoption. Research from the Infosys Bank Tech Index: Volume 3 shows that both these technologies account for less than 5% of banking technology budgets and recruitment.
AI: A hype or reality
With the consumerization of AI, through the likes of ChatGPT, individuals have started experiencing AI at a personal level, which is reminiscent of the mobile phone revolution. Bulky landline phones were replaced by feature phones, and those gave way to smartphones. AI is likely to have the same journey but at a much faster pace. Additionally, enterprise adoption of AI has increased. The technology now accounts for 31% of bank technology budgets as per the Infosys Bank Tech Index, up from 20% previously.
But how do we know that the recent push in AI is not just hype? There are three factors to consider if the spark of a technology is short-lived or it is at the crest of a new era.
1. Business value is emerging
Emerging research shows that AI could boost revenues in the banking sector, and research from the Infosys Knowledge Institute also shows that financial institutions are ahead of the curve when it comes to generating business value from AI, with 16% reporting that they had done so compared to the average of other industries at 13%.
But it is still early, and much of the industry is still experimenting. Use cases range from software development and managing adverse media (Deutsche Bank) to analyzing Federal Reserve speeches and detecting fraud (JPMorgan) and even personalized financial advice and recommendations (Morgan Stanley).
These use cases are capturing investors’ attention: generative AI spending by financial institutions is expected to rise 164% in 2024. By 2026, global AI spending on systems, services, and platforms will be $300 billion according to IDC, and the financial services industry is expected to hold the lion’s share.
2. Positive societal impact
As an example, digital transformation in India has led to increased financial inclusion, with nearly 78% of the population having access to banking – up from just 35% a decade ago.
But, there is still work to do here. Many people still struggle to use mobile banking applications due to literacy challenges, language barriers, age, or physical impairments. AI can support these groups with voice-activated banking as well as personalized financial advice. AI can also be used to derive the creditworthiness of the unbanked populace by analyzing alternative data for credit scoring, especially for those with limited to no credit history. This will help drive microcredit while boosting financial inclusion.
3. Regulations are catching up
Regulatory compliance is paramount for financial institutions looking to employ AI and go AI-first. But, the tech is moving so fast that most regulators are still playing catch-up. While this is worrying, work is being done to respond to concerns about bias, discrimination, and other ethical nightmares that come with improper use of the technology. Even as the regulatory environment for AI is still evolving, governments and regulatory watchdogs have begun introducing regulations – the US government’s Executive Order, the EU AI Act, and the UK’s Guidelines for Secure AI System Development – so firms can build ethical, responsible AI.
Institutions, on their part, must integrate ethical considerations into the design and architecture by developing a responsible design framework for ethical AI usage. For example, in the mortgage or credit underwriting process, regulators require an audit trail and information must be logged on why the decision was made, what parameters were considered, and were decisions made without any bias. With the right systems in place, AI can make better, faster, and less erroneous decisions than a human. AI can eliminate inherent human bias, making decisions in an ethical and responsible manner.
The challenges that hold technologies back
Enterprises face various hurdles when it comes to AI adoption. The technologies that make AI so powerful are the cloud and data. The cloud offers a dynamic and efficient foundation by enabling access to computing resources, storage, and innovative services. Data is key for financial institutions to train AI models. Yet institutions face hurdles in the form of data quality, accessibility, and governance. Ensuring that their AI systems do not violate privacy, prevent bias from creeping in, and remain secure keeps enterprise CXOs awake at night.
Sustainable energy consumption, costs and regulations are also challenges when it comes to generative AI. These were among the many challenges that made blockchain and metaverse unviable.
It is different with generative AI. Regulators, although slow, are more forthcoming with regulations. Large language models have given way to the emergence of focused and specific narrow transformers, making energy and costs sustainable. The right talent is the bedrock for building resilient, compliant, and secure AI systems. Yet, the Infosys Bank Tech Index found that AI and cybersecurity talent are the most difficult skills for enterprises to recruit.
What does the future hold for AI?
The interesting dichotomy with AI is that it can automate hacking, sidestepping traditional security – yet it can also bolster security through anomaly detection, threat prediction, and real-time monitoring. Institutions must continuously adapt to stay ahead of risks, which can shape the industry’s future by addressing data privacy, integrity, and fairness.
While the jury is still out as to who will win between skeptics and futurists, we believe that in five years, AI will solve complex business problems – and do it at scale.The tech still has a long way to go as it is faced with many challenges. AI isn’t yet providing early warning signals in risk, while fraud and cyber threats continue to rise.It also needs to improve decision-making for portfolio analysis and for making credit decisions. While the tech will become mainstream across financial institutions, now is the time to put together an integrated AI-first led strategy and experiment and evolve to build competitive differentiation and realize the bank’s business objectives.
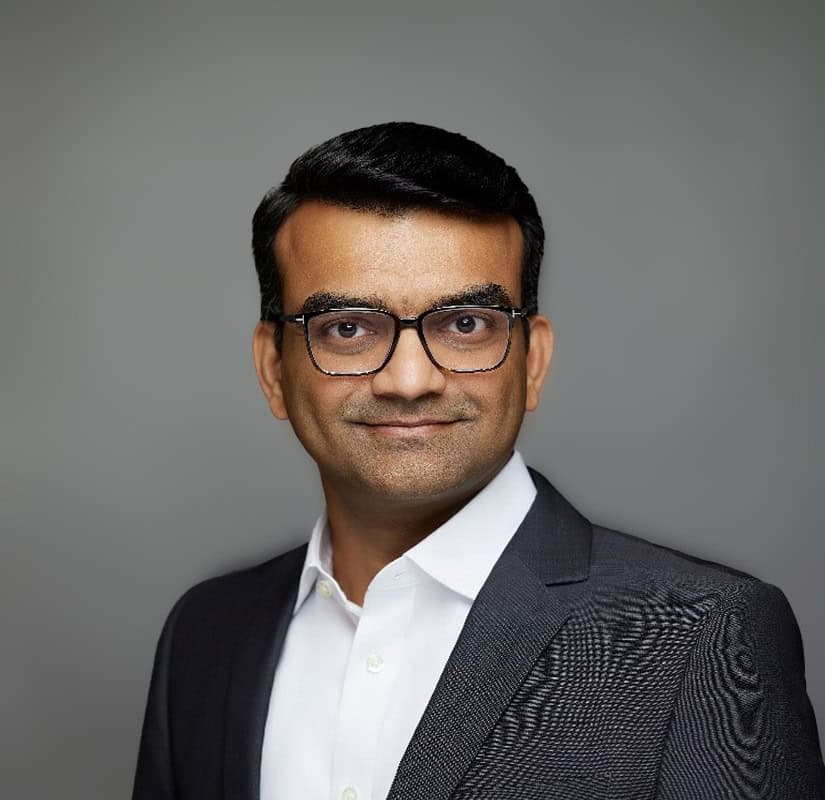
Dennis Gada
Executive Vice President and Global Head of Banking & Financial Services | Infosys
As the Global Head of Banking & Financial Services at Infosys, Dennis leads the largest business unit within Infosys along with his Global Financial Services Executive Leadership team. He is a Board Member of EdgeVerve Systems Limited, a Products and Platform subsidiary of Infosys and Infosys Compaz Pte. Ltd (iCompaz) a Joint Venture between Infosys Limited and Temasek Holdings. In his current role, Dennis is responsible for strategic direction, growth, operational excellence, and all commercial and fiscal management of the Global Banking & Financial Services business. With more than two decades of professional experience living and working across APAC, Europe, and the US, Dennis currently resides in Charlotte, North Carolina with his family.
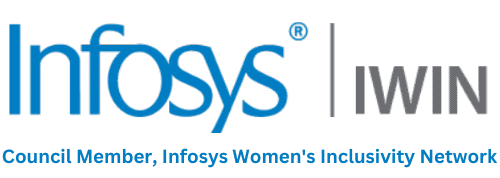